Connectomics: Understanding the Human Existence
- Alondra Sánchez
- Jan 14
- 11 min read
Written by: Alondra Sánchez
Edited by: Isabella Polsfuss and Penelope Figueroa
Illustrated by: Mina Kamara
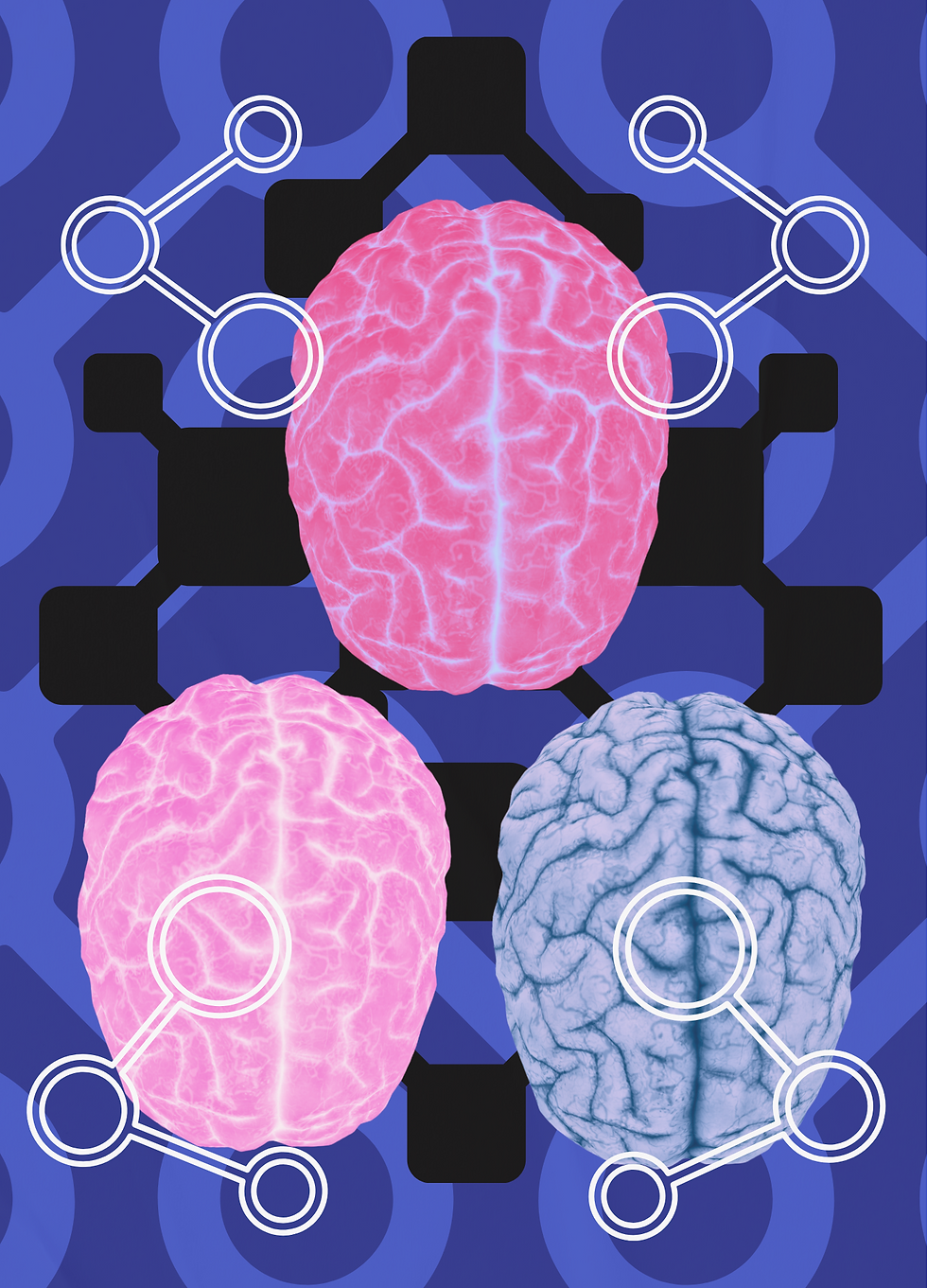
Introduction
In 1909, the German neuroscientist Korbinian Brodmann was credited with having laid the foundation of modern neuroscience with his creation of the Brodmann map [1]. This revolutionary map divided the human cerebral cortex into 52 distinct regions [2]. The cerebral cortex is a network of neurons that spans over the brain’s outer layer, organized into four lobes (frontal, occipital, parietal, and temporal), each serving unique roles [3]. Brodmann’s pioneering work represented a significant advancement in tackling the core question: how many distinct parts constitute the brain? In 2010, the Human Connectome Project (HCP) ushered in a new era of exploration into this inquiry. Harnessing state-of-the-art technologies and vast data collection capabilities, the HCP unveiled an astounding 180 regions within the human cerebral cortex, 97 of which were newly discovered [4]. To this day, efforts to further identify new regions in the brain are being made. The intricate complexity and study of the human brain requires the use of advanced systems for its analysis, and attempting to simplify it for convenience is impractical. Thus, a field of study, called connectomics, involves handling extensive brain-related data in an aim to make the information understandable and easier to evaluate. In other words, connectomics refers to the effort focused on creating a thorough map of the entire brain. However, in order to understand the role of connectomics in neuroscience, it is important to first look at its practical applications such as in neurosurgery.
Connectomics
Connectomics uses magnetic resonance imaging (MRI) tools such as diffusion MRI (dMRI), a technique used to examine the flow and direction of water in the brain’s white matter [5]. White matter encompasses the brain region composed of nerve fibers that facilitate the exchange of information and communication among various brain areas [6]. A dMRI scan can be represented by a three-dimensional modeling technique called tractography, which makes it possible to visualize these nerve tracts [5]. Using dMRI and tractography, neurosurgeons are able to create a map of a patient’s brain before surgery and locate surgical targets such as brain tumors [7]. This enables neurosurgeons to formulate a tailored preoperative strategy for each patient [8]. In a 2023 study, postoperative MRIs showed that with the help of connectomics, surgeons were able to successfully remove a tumor and the impacted tissue that surrounded it with no signs of postoperative problems [9].
As neurosurgeons navigate the landscape of a patient’s brain to locate tumors, they simultaneously identify specific brain regions. These areas represent predetermined clusters of brain tissues, each with its own distinct functions and contributions to overall cognitive processes [8]. Examples of these areas include the hippocampus (responsible for memory formation), the amygdala (involved in processing emotions), and the visual and motor cortices (which handle visual perception and voluntary movement) [10]. By integrating connectomics into surgical procedures, neurosurgeons can maneuver around these areas during the removal (also known as resection) of a tumor, minimizing the risk of unintended damage [8]. On the other hand, without the help of connectomics, there exists a heightened risk of disrupting or removing essential areas potentially leading to detrimental outcomes such as the loss of sensation [8]. Beyond its effectiveness in the postoperative phase, connectomics was initially conceived with the philosophy of overcoming barriers posed by inoperable tumors, thereby enabling surgical resections in specific brain regions previously deemed too hazardous and inaccessible [11].
Limitations
Given that connectomics is still in its infancy, several issues underscore the imperative need for further research to bolster the promising potential of this field. One significant concern is the reliability of connectomics data, as tractography occasionally generates misleading results by suggesting the presence of neural connections that do not actually exist [12]. However, these erroneous technical findings may also hint at the existence of another invisible “network” yet to be discovered. As tractography algorithms continue to improve through the use of machine learning (when computers interpret big data to identify patterns and to teach and make their own recommendations or decisions [13]), the nerve tracts they show may change [7]. Besides improving algorithmic outputs (the result after processing the input of data), there also exists a need to enhance the usability of preoperative images after intraoperative changes have been made. To improve surgeries and results, it’s important to deal with changes that happen during surgery itself. These changes can make the connectomic maps made before surgery useless since the brain is altered in real-time during the operation [14]. Factors such as the opening of the skull to the brain, the size of brain tissue removal, and the presence of brain swelling are known to play a role in influencing the dynamic shifts in the patient’s connectomic map during surgery [7]. Hence, it is essential to incorporate constant updates of intraoperative remapping and real-time imaging to optimize their utility [7]. This motivates researchers to assess the dependability of connectomic applications in surgical contexts at a clinical level, rather than solely from an algorithmic perspective [7].
In order for connectomics to become recognized as an undoubtedly reliable neurosurgical tool, clinically relevant research must be conducted [15]. There’s limited research on using connectomics data in clinical settings, such as understanding how diseases change brain maps or using connectomics to decide on treatments [16]. To gain a thorough understanding of the true impact of connectomics on enhancing surgical outcomes, it is imperative to consider and assess the patient’s entire neurosurgical experience. This encompasses various factors, including the effectiveness of the surgical procedure itself, the patient’s recovery process, the patient’s postoperative satisfaction, any postoperative complications, and long-term outcomes such as quality of life and neurological function [17]. Evaluating the patient’s neurosurgical experience provides valuable insights into how connectomics interventions integrate with and influence the overall surgical process, ultimately contributing to a more holistic assessment of their benefits and limitations. While clinicians and physicians assess the clinical applications of connectomics, researchers can explore various algorithms and additional features to enhance connectomics, ultimately contributing to improving its reliability.
Future Outlooks
Considering the complexity of the human brain, it’s no surprise that medical professionals are still cultivating a coherent connectome model. Connectome refers to a representation of the brain’s structural connections presented as a network [18]. Researchers have used data and images from MRI scans to create detailed three-dimensional diagrams of individual neurons. However, the hundreds of MRI images taken daily only show cellular connections and neural tissue at a microscopic scale, down to the nanometer [19]. Additionally, due to the low pixel density in 30-nanometer slices of brain images, creating a one-millimeter brain section requires a huge amount of storage (62.5 gigabytes) for every single image [19]. Given this, researchers predict it would take them up to 600 years to finish mapping a small rodent’s cerebral cortex connectome, which is just 0.1% the size of a human’s cerebral cortex [19]. These rates of acquiring neural images is not feasible, especially when moving to the human brain [19]. This calls for new imaging microscopes that can use multiple ways to map each neuron at a larger and faster scale [19]. The question shifts from “can we map the brain?” to “how fast can we map the entire brain?”
Given the immense and ongoing daily data generation, the human brain connectome represents one of larger data sets in the world. This highlights an urgent requirement for substantial expansion in data storage capacity and a discussion regarding the methods to achieve this become particularly important. Mass univariate analysis refers to a method of studying data by doing lots of statistical tests and comparing many pieces of information to understand patterns in the data [20]. This method is helpful when researchers lack precise information about the data they’re studying, and in relation to connectomics, researchers might consider using this method to map out unknown areas of the brain. However, the complexity of a connectome suggests moving away from this traditional data-driven approach [21] to a more advanced approach such as utilizing machine learning (ML), where computers gain the capability to learn independently without the need for continuous and explicit programming [22]. With its current use in connectomics, ML allowed researchers to analyze how each nanometer section of an MRI image relates to the overall brain MRI scan [21], thus helping to identify brain regions. ML can also recognize intricate changes in brain structure and function caused by disease or medications [21] that mass univariate analysis is not capable of doing. Connectomics also uses deep learning (DL) algorithms, which are specialized software tools that help create and use networks more efficiently [23]. The combination of machine learning and deep learning algorithms aids the successful production of connectomes [24].
Aside from using ML to speed up the process of data mapping, and DL to precisely identify unknown brain regions, another aspect that can potentially upgrade the creation of a connectome is using a tool to better physically visualize the representation of big brain data. This invites the form of visually interacting with the collected data where researchers could perceive it in a three-dimensional format. In other words, the human brain map could lie in the hands of augmented reality (AR). AR is a technology that lays digital information (images, videos, three-dimensional models) on top of the real-world environment which improves how a user sees or understands something [25]. In fact, AR has already been used to improve neurosurgery navigation which allows surgeons to know the location of their target and the path to reach their target safely [26]. During 2000 and 2002, the Women’s Medical University in Japan conducted a study that followed 85 patients who underwent brain tumor surgery where surgeons used AR to navigate their operation [27]. The results showed that with the help of AR, surgeons were able to increase the amount of tumor removal from 95% to 100%, and the patient’s five-year survival prediction rose from 22.5% to 40.9% [28]. By showcasing that AR improves the duties of surgeons, AR represents the benefits of its meld with connectomics. A tool that uses similar aspects of AR in a more engaging and entertaining manner is Unreal Engine. Unreal Engine is a computer program that can detect when objects collide, think on its own (artificial intelligence), see what’s around it (visibility), connect to other devices or systems (networking), follow instructions (scripting), and manage files [29]. This software enables the “agent” or “player” to interact and move around in life-like virtual environments [30]. There are currently no studies being conducted that incorporate the use of Unreal Engine to aid the creation of a connectome. However, given the characteristics of this computer program, Unreal Engine could potentially revolutionize connectomics by programming data to visually represent how and when neural tracts interact, where neural tracts lead to, how different brain regions work together, and might help to visually trace changes of the brain that don’t appear otherwise in a scan. It could also tackle challenges such as data storage and time constraints in connectomics by translating all the MRI and tractography data into a virtual world.
Conclusion
Connectomics has traversed a remarkable journey, and it’s imperative to honor its origins with Dr. K. Brodmann, whose foundational work set the stage. From initially identifying fewer than 60 brain regions to envisioning its meld with an interactive computer program, connectomics presents an exciting trajectory for advancing our understanding of the human brain. With a comprehensive brain map, surgeons can enhance surgical precision across preoperative, intraoperative, and postoperative stages for positive surgical outcomes and patient survival success. Beyond its medical applications, connectomics opens doors for the development of coding analysis by computer programmers, statistical exploration by statisticians, and even creative insights for graphic designers. Moreover, connectomics demonstrates that questions once we thought were impossible to answer can now be seen as questions about how accurately and precisely we can get the answers we’re looking for. This interdisciplinary effort isn’t limited to the medical field; it’s a global endeavor that enriches our understanding of human existence.
References
[1] Loukas, M., et al. (2011). Korbinian Brodmann and His Contributions to Mapping the Cerebral Cortex. Neurosurgery, 68, 6-11.
[2] Annese, J. (2009). In Retrospect: Brodmann's brain map. Nature, 461, 884. https://doi.org/10.1038/461884a.
[3] Javed K., Reddy V., Lui F. (2023). Neuroanatomy, Cerebral Cortex. StatPearls Publishing. https://www.ncbi.nlm.nih.gov/books/NBK537247/
[4] Glasser, M. F., Coalson, T. S., Robinson, E. C., Hacker, C. D., Harwell, J., Yacoub, E., Ugurbil, K., Andersson, J., Beckmann, C. F., Jenkinson, M., Smith, S. M., & Van Essen, D. C. (2016). A multi-modal parcellation of human cerebral cortex. Nature, 536(7615), 171–178. https://doi.org/10.1038/nature18933
[5] Toga, A. W., Clark, K. A., Thompson, P. M., Shattuck, D. W., & Van Horn, J. D. (2012). Mapping the human connectome. Neurosurgery, 71(1), 1–5. https://doi.org/10.1227/NEU.0b013e318258e9ff
[6] Filley, C. & Fields, R. (2016). White matter and cognition: making the connection. Journal of Neurophysiology, 116 (5), 2093 - 2104. https://doi.org/10.1152/jn.00221.2016
[7] Yang, J., et al. (2021) Diffusion MRI tractography for neurosurgery: the basics, current state, technical reliability and challenges. Phys. Med. Biol. 66 15TR01
[8] Hart, M. G., Price, S. J., & Suckling, J. (2016). Connectome analysis for pre-operative brain mapping in neurosurgery. British Journal of Neurosurgery, 30(5), 506–517. https://doi.org/10.1080/02688697.2016.1208809
[9] Suero Molina, E., Tait, M. J., & Di Ieva, A. (2023). Connectomics as a prognostic tool of functional outcome in glioma surgery of the supplementary motor area: illustrative case. Journal of neurosurgery. Case lessons, 6(6), CASE23286. https://doi.org/10.3171/CASE23286
[10] Watson, C., Kirkcaldie, M., & Paxinos, G. (2010). The brain an introduction to functional neuroanatomy. Elsevier.
[11] Duffau, H. (2014). Surgical Neurooncology Is a Brain Networks Surgery: A “Connectomic” Perspective. World Neurosurgery, 82, 405-407. https://doi.org/10.1016/j.wneu.2013.02.051.
[12] Maier-Hein, K.H., Neher, P.F., Houde, JC. et al. The challenge of mapping the human connectome based on diffusion tractography. Nat Commun 8, 1349 (2017). https://doi.org/10.1038/s41467-017-01285-x
[13] Helm, J.M., Swiergosz, A.M., Haeberle, H.S. et al. Machine Learning and Artificial Intelligence: Definitions, Applications, and Future Directions. Curr Rev Musculoskelet Med 13, 69–76 (2020). https://doi.org/10.1007/s12178-020-09600-8
[14] Hayashi, Y., et al. (2014). Functional Reorganization in the Patient with Progressing Glioma of the Pure Primary Motor Cortex: A Case Report with Special Reference to the Topographic Central Sulcus Defined by Somatosensory-Evoked Potential.
World Neurosurgery, 82, 536.
[15] Henderson, F., Abdullah, K., et al. (2020). Tractography and the connectome in neurosurgical treatment of gliomas: the premise, the progress, and the potential. Journal of Neurosurgery, 48 (2). https://doi.org/10.3171/2019.11.FOCUS19785
[16] Shah, H., Mehta, N., et al. (2022). Connecting the connectome: A bibliometric investigation of the 50 most cited articles. Clinical Neurology and Neurosurgery, 223, 107481.
[17] Jaensson, M., Dahlberg, K., & Nilsson, U. (2019). Factors influencing day surgery patients' quality of postoperative recovery and satisfaction with recovery: a narrative review. Perioperative medicine (London, England), 8, 3. https://doi.org/10.1186/s13741-019-0115-1
[18] Sporns, O. (2013). The human connectome: Origins and challenges. NeuroImage, 80,
[19] Lichtman, J. W., Pfister, H., & Shavit, N. (2014). The big data challenges of connectomics. Nature neuroscience, 17(11), 1448–1454. https://doi.org/10.1038/nn.3837
[20] Groppe, D., et al. (2011). Mass univariate analysis of event-related brain potentials/fields I: A critical tutorial review. Psychophysiology, 48 (12), 1711-1725. https://doi.org/10.1111/j.1469-8986.2011.01273.x
[21] Ktena, S., Sotiras, A., et al. (2023). Chapter 12 - Machine learning in connectomics: from representation learning to model fitting. Connectome Analysis, 267-287.
[22] Mahesh, B. (2018). Machine Learning Algorithms -A review. International Journal of Science and Research. https://www.researchgate.net/publication/344717762_Machine_Learning_Algorithms_-A_Review
[23] Deep learning. Deep Learning - an overview | ScienceDirect Topics. (n.d.). https://www.sciencedirect.com/topics/engineering/deep-learning
[24] Dvornek, N. & Li, X. (2023). Chapter 13 - Deep learning with connectomes,
Connectome Analysis, 289-308. https://doi.org/10.1016/B978-0-323-85280-7.00013-0.
[25] Carmigniani, J., Furht, B. (2011). Augmented Reality: An Overview. In: Furht, B. (eds) Handbook of Augmented Reality. Springer, New York, NY. https://doi.org/10.1007/978-1-4614-0064-6_1
[26] Mezger, U., Jendrewski, C. & Bartels, M. Navigation in surgery. Langenbecks Arch Surg 398, 501–514 (2013). https://doi.org/10.1007/s00423-013-1059-4
[27] Iseki, H., Muragaki, Y., et al. (2003). Clinical application of augmented reality in neurosurgical field. Proceedings Computer Graphics International, 44-49.
[28] Dadario, N., Quinoa, T., et al. (2021). Examining the benefits of extended reality in neurosurgery: A systematic review. Journal of Clinical Neuroscience, 94, 41-53. https://doi.org/10.1016/j.jocn.2021.09.037.
[29] Sanders, A. (2016). An Introduction to Unreal Engine 4 (1st ed.). A K Peters/CRC Press. https://doi.org/10.1201/9781315382555
[30] Qiu, W., Yuille, A. (2016). UnrealCV: Connecting Computer Vision to Unreal Engine. In: Hua, G., Jégou, H. (eds) Computer Vision – ECCV 2016 Workshops. ECCV 2016. Lecture Notes in Computer Science(), vol 9915. Springer, Cham. https://doi.org/10.1007/978-3-319-49409-8_75
Comments