Balancing Innovation and Access: Magnetic Resonance Imaging, Artificial Intelligence, Patent Law, and the Future of Equitable Healthcare
- Jessica Nwosu
- Jan 23
- 9 min read
Writtten by: Jessica Nwosu
Edited by: Hiuyi Cheng, Sherry Guo, and Shivani Patel
Illustrated by: Elvan Eren
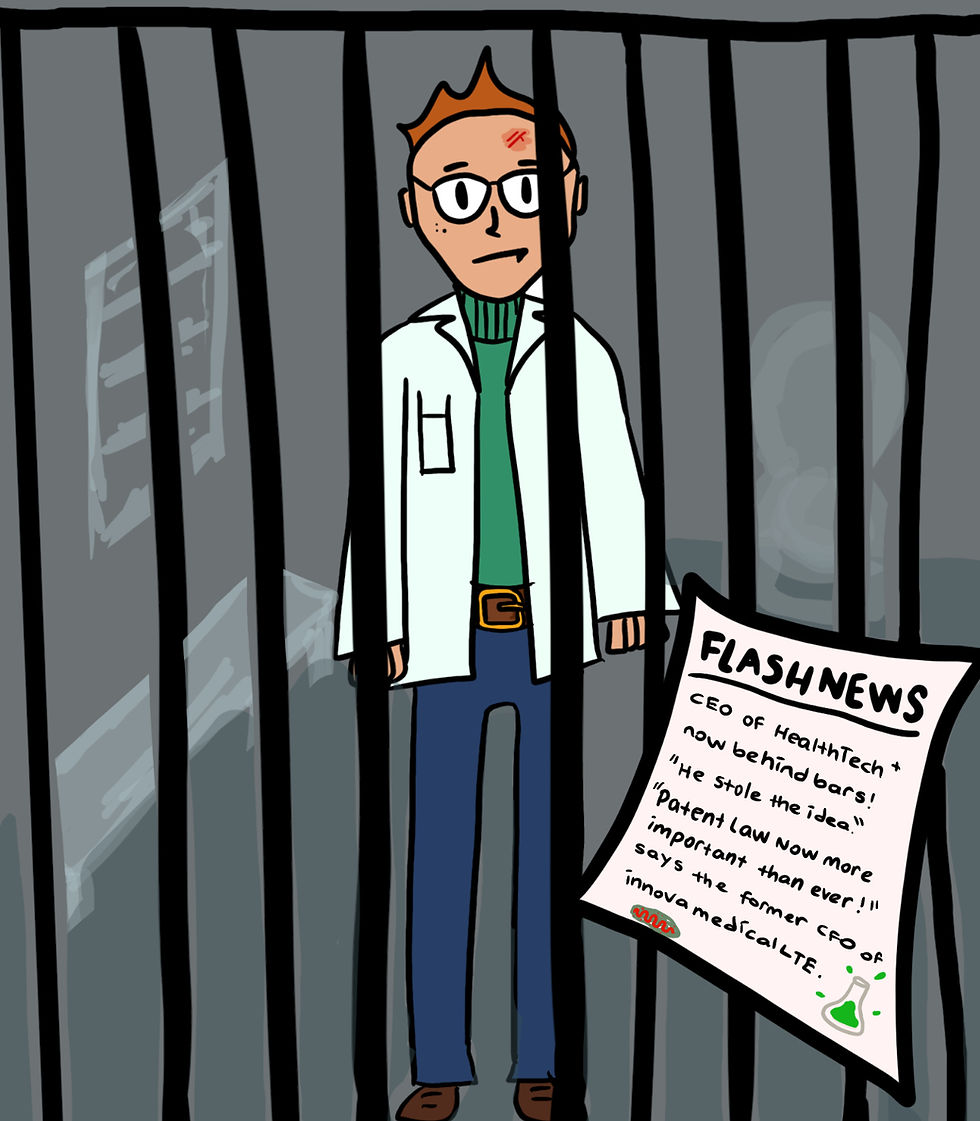
Healthcare has entered an era of rapid transformation driven by advancements in medical imaging, artificial intelligence (AI), and patent law. Each of these elements plays a crucial role in shaping how we diagnose and treat diseases, but they also introduce unique challenges. Medical imaging, particularly magnetic resonance imaging (MRI) technology, has evolved significantly due to AI's ability to enhance diagnostic precision and efficiency. Meanwhile, patent law incentivizes innovation but can also restrict access to cutting-edge technologies. These intertwined factors, compounded by political influences like the US Food and Drug Administration (FDA) regulations and insurance policies, create a complex landscape that raises important questions about the future of equitable healthcare. Understanding these dynamics is essential to evaluating whether these innovations will bridge healthcare gaps or deepen existing disparities.
In recent years, medical imaging, particularly MRI technology, has seen significant advancements propelled by AI and data science. For example, according to Shimron et al., AI has propelled MRI use in hospitals through image synthesis and parameter quantification—the ability to derive meaningful tissue-characterizing images from raw data [1]. These tools significantly enhance MRI’s role as a cornerstone of modern diagnostics by providing faster, more accurate, and detailed insights into various medical conditions, from neurological disorders to cancer [2]. By leveraging AI and advanced data science, MRI can detect subtle anomalies that might otherwise be missed, enabling earlier and more precise interventions [2]. For instance, in neurological conditions like Alzheimer’s disease, AI-enhanced MRI can identify early-stage biomarkers in MRI scans, allowing for timely treatment that may slow disease progression [3]. Similarly, in oncology, advanced MRI techniques can distinguish between benign and malignant tumors with greater confidence, reducing the need for invasive biopsies, and guiding more targeted treatment plans [4]. The ability to deliver such accurate and efficient diagnostics not only improves patient outcomes but also streamlines healthcare processes, reducing diagnostic errors, minimizing costs, and ultimately enhancing the quality of care across medical disciplines [5].
As healthcare innovation becomes increasingly complex, regulations must balance incentivizing new technologies with ensuring access to essential healthcare tools. This challenge is compounded by the political landscape, where FDA regulations, insurance policies, and lobbying efforts shape which medical innovations reach the market and who can afford them. In exploring the intersections of MRI technology, AI, and patent law, this article examines how these forces collectively influence the future of healthcare, particularly in terms of equity and accessibility.
MRI and Data Science in Modern Healthcare
Magnetic Resonance Imaging and Artificial Intelligence
While AI has proven to be a powerful tool in enhancing MRI diagnostics, it is not a substitute for human expertise [6]. Physicians remain an integral part of the diagnostic process, as they bring clinical context and judgment that AI lacks. Radiologists and other specialists carefully review AI-assisted scans to verify findings, assess anomalies, and ensure accuracy before making final decisions. This collaborative approach combines the efficiency of AI with the critical thinking and experience of medical professionals, ensuring patient safety and optimal outcomes.
This synergy between AI and human expertise is particularly evident in one of the most promising advancements in MRI technology: the integration of Artificial Intelligence. Traditional MRI scans, while effective, can be time-consuming and require highly skilled interpretation. AI applications, by contrast, can analyze vast amounts of MRI data using machine learning algorithms trained on extensive datasets of medical images [7]. These algorithms identify patterns by comparing new scans to known cases, detecting anomalies, and learning to differentiate between normal and abnormal structures. For example, convolutional neural networks (CNNs), a type of deep learning model, excel at identifying intricate features within MRI images, enabling the precise differentiation between benign and malignant lesions. This rapid, data-driven analysis enhances diagnostic accuracy, providing radiologists with a powerful tool to support clinical decision-making.
Data Privacy and Ownership
The integration of AI in MRI comes with significant data requirements because large datasets are necessary to train algorithms effectively [8]. This reliance on data brings challenges related to patient privacy. Patient data, often gathered across multiple platforms, is invaluable for training AI systems, yet concerns over who owns and controls this data are growing. Many patients are uneasy with the idea of their health data being shared or sold, especially without explicit consent [9].
In addition, data ownership raises questions about the equitable distribution of AI. Large medical institutions and corporations have greater access to extensive datasets, enabling them to develop and patent AI-based MRI tools more readily than smaller entities [10]. This imbalance could lead to disparities in access, as only well-funded institutions may be able to afford or develop such technology.
The Role of Patents in Innovation
Patents and Medical Technology
Patent law is crucial to the development of medical technology, offering inventors exclusive rights to their creations, thereby incentivizing innovation [11]. By ensuring innovators are rewarded for their labor and investment, patents play a vital role in advancing high-stakes fields like healthcare [11]. For example, IBM's patent for "Method and System for Optimized Medical Diagnosis Based on Machine Learning" exemplifies how patent protection fosters innovation in AI-powered medical diagnostics [12]. In the context of MRI technology and AI, patents like this help companies justify the costs associated with research and development, enabling them to recoup investments by safeguarding proprietary algorithms, hardware improvements, and other groundbreaking innovations [13].
Barriers to Access
Patents, while crucial for incentivizing innovation, can also create barriers to access. When MRI or AI technologies are patented, the patent holder may impose high licensing fees, restricting their use to wealthier institutions and delaying the adoption of groundbreaking diagnostic tools in underfunded hospitals and healthcare systems. This contributes to systemic disparities in healthcare, where some hospitals have access to state-of-the-art AI-enhanced MRI machines while others rely on older, less effective technologies [14].
Disparities in diagnostic capabilities often stem from systemic issues such as resource inequities, outdated equipment, and inadequate follow-up systems [15]. According to the Agency for Healthcare Research and Quality, hospitals utilizing only standard X-ray or ultrasound imaging for complex conditions, such as cancer or neurological disorders, may miss abnormalities detectable by advanced MRI. Such limitations often lead to delayed or missed diagnoses, worsening health outcomes for patients in resource-limited settings.
Moreover, patent protections can stifle innovation by preventing other researchers from building on patented work, raising concerns about balancing rewards for innovation with ensuring accessibility to life-saving technologies [16]. Reforming patent law to consider both innovation and equity could help address these disparities, ensuring advanced tools like AI-enhanced MRI are more widely available.
Socio-economic and political factors further exacerbate these inequalities. Wealthier institutions are more likely to afford advanced MRI technology, while underfunded hospitals and marginalized communities, often lacking the political influence to advocate for better resources, remain reliant on outdated tools [5]. This cycle perpetuates healthcare inequities, as vulnerable populations face reduced access to timely and accurate diagnostics. Public health policies, influenced by political priorities rather than need, often lead to unequal resource distribution, leaving rural and underserved areas at a significant disadvantage [5]. Addressing these systemic issues is critical to ensuring equitable healthcare access and improving diagnostic safety for all.
The Political Influence on Healthcare Access
Regulations and Policies
The accessibility of MRI and AI innovations is deeply influenced by political forces, particularly FDA regulations, insurance policies, and lobbying. The FDA plays a critical role in determining which medical technologies reach the public by setting safety and efficacy standards [17]. However, this regulatory process can be time-consuming and costly, deterring smaller companies or research institutions from entering the market [18]. While these regulations are essential for patient safety, they can hinder the swift introduction of beneficial technologies.
Insurance policies further complicate access. Even when the FDA approves a new diagnostic tool, insurance companies may not cover its use, making it prohibitively expensive for many patients. For example, a hospital might offer AI-enhanced MRI scans that can detect subtle early-stage abnormalities, such as small tumors or microvascular changes, with remarkable precision. However, if insurance does not cover the additional cost of this advanced technology, patients may be forced to opt for traditional imaging methods, such as X-rays or standard ultrasounds. While these alternatives are valuable diagnostic tools, they may be less effective at detecting fine details in soft tissues, leading to delayed or missed diagnoses, particularly in cases like early-stage cancers or neurological conditions [10].
One real-world example is the use of mammography versus a breast MRI in cancer detection. While mammograms are a standard and widely utilized method, breast MRI is more sensitive in detecting cancers in high-risk patients, particularly those with dense breast tissue [5]. Studies have shown that breast MRI can identify cancers that mammograms may miss. However, the high cost and limited insurance coverage for breast MRI often force patients to rely solely on mammography, increasing the risk of late-stage diagnoses [19]. This disparity underscores how insurance policies and cost barriers can dictate the quality of diagnostic care patients receive.
Lobbying by powerful corporations and insurance providers significantly impacts the adoption and accessibility of healthcare innovations, often delaying or redirecting the adoption of advanced diagnostic tools based on financial or strategic priorities. Well-funded lobbying campaigns can delay or prevent the widespread adoption of costlier AI-enhanced tools in favor of more traditional, less expensive alternatives. These efforts shape regulatory frameworks and funding decisions, prioritizing profitability over patient outcomes, leaving patients and underfunded healthcare systems with fewer options, and perpetuating disparities in access to cutting-edge medical advancements [20]. As a result, the political and financial priorities of lobbying entities significantly shape the healthcare landscape, creating controversy about access to healthcare solutions.
Conclusion
In the future, the convergence of MRI technology, AI, patent law, and political influence will shape the future of healthcare in profound ways. Advancements in MRI and AI have the potential to revolutionize diagnostics, offering unprecedented accuracy and efficiency. Nevertheless, these technologies raise issues related to privacy, ownership, and accessibility as they intersect with patent law and regulatory policies.
The future of healthcare will likely depend on how well these challenges are addressed. Policymakers, healthcare professionals, and innovators must collaborate to ensure that technological advancements benefit all regions of the population, not just those who can afford them. Patent law reforms that balance innovation with accessibility, alongside policies that prioritize equitable healthcare distribution, will be essential in creating a healthcare system that serves all.
References
[1] Shimron, E., & Perlman, O. (2023). AI in MRI: Computational Frameworks for a Faster, Optimized, and Automated Imaging Workflow. Bioengineering (Basel, Switzerland), 10(4), 492. https://doi.org/10.3390/bioengineering10040492
[2] Fass, L. (2008). Imaging and cancer: A review. Molecular Oncology, 2(2), 115–152. https://doi.org/10.1016/j.molonc.2008.04.001
[3] Maleki Varnosfaderani, S., & Forouzanfar, M. (2024). The role of AI in hospitals and clinics: Transforming healthcare in the 21st century. Bioengineering (Basel, Switzerland), 11(4), 337. https://doi.org/10.3390/bioengineering11040337
[4] Bi, W. L., Hosny, A., Schabath, M. B., Giger, M. L., Birkbak, N. J., Mehrtash, A., ... & Aerts, H. J. W. L. (2019). Artificial intelligence in cancer imaging: Clinical challenges and applications. CA: A Cancer Journal for Clinicians, 69(2), 127–157. https://doi.org/10.3322/caac.21552
[5] Salem, D. S., Kamal, R. M., Mansour, S. M., Salah, L. A., & Wessam, R. (2013). Breast imaging in the young: The role of magnetic resonance imaging in breast cancer screening, diagnosis, and follow-up. Journal of Thoracic Disease, 5(Suppl 1), S9–S18. https://doi.org/10.3978/j.issn.2072-1439.2013.05.02
[6] Najjar R. (2023). Redefining Radiology: A Review of Artificial Intelligence Integration in Medical Imaging. Diagnostics (Basel, Switzerland), 13(17), 2760. https://doi.org/10.3390/diagnostics13172760
[7] Khalifa, M., & Albadawy, M. (2024). AI in diagnostic imaging: Revolutionising accuracy and efficiency. Computer Methods and Programs in Biomedicine Update, 5, 100146. https://doi.org/10.1016/j.cmpbup.2024.100146
[8] National Academies of Sciences, Engineering, and Medicine, Health and Medicine Division, Board on Population Health and Public Health Practice, & Committee on Community-Based Solutions to Promote Health Equity in the United States. (2017). Baciu, A., Negussie, Y., Geller, A., & Weinstein, J. N. (Eds.). Communities in action: Pathways to health equity. National Academies Press. https://www.ncbi.nlm.nih.gov/books/NBK425845/
[9] Cascini, F., Pantovic, A., Al-Ajlouni, Y. A., Puleo, V., De Maio, L., & Ricciardi, W. (2024). Health data sharing attitudes towards primary and secondary use of data: A systematic review. EClinicalMedicine, 71, 102551. https://doi.org/10.1016/j.eclinm.2024.102551
[10] Khalifa, M., & Albadawy, M. (2024). AI in diagnostic imaging: Revolutionising accuracy and efficiency. Computer Methods and Programs in Biomedicine Update, 5, 100146. https://doi.org/10.1016/j.cmpbup.2024.100146
[11] Pinto-Coelho, L. (2023). How artificial intelligence is shaping medical imaging technology: A survey of innovations and applications. Bioengineering (Basel, Switzerland), 10(12), 1435. https://doi.org/10.3390/bioengineering10121435
[12] IBM. (2020). Method and system for optimized medical diagnosis based on machine learning (U.S. Patent No. 10,534,778). United States Patent and Trademark Office. Retrieved from https://patents.google.com/patent/US10534778B2
[13] Saha, C. N., & Bhattacharya, S. (2011). Intellectual property rights: An overview and implications in the pharmaceutical industry. Journal of Advanced Pharmaceutical Technology & Research, 2(2), 88–93. https://doi.org/10.4103/2231-4040.82952
[14] Liu, S., & Guo, L. R. (2024). Data ownership in the AI-powered integrative health care landscape. JMIR Medical Informatics, 12, e57754. https://doi.org/10.2196/57754
[15] Mirkin, S., & Albensi, B. C. (2023). Should artificial intelligence be used in conjunction with neuroimaging in the diagnosis of Alzheimer’s disease? Frontiers in Aging Neuroscience, 15, 1094233. https://doi.org/10.3389/fnagi.2023.1094233
[16] Cypris. (n.d.). Do patents stifle innovation? Everything you need to know. Retrieved January 4, 2025, from https://www.cypris.ai/insights/do-patents-stifle-innovation-everything-you-need-to-know
[17] The Pew Charitable Trusts. (2021, August). How FDA regulates artificial intelligence in medical products. Retrieved from https://www.pewtrusts.org/en/research-and-analysis/issue-briefs/2021/08/how-fda-regulates-artificial-intelligence-in-medical-products
[18] Reiss, D. (2021, June 14). Politicization of science. American Bar Association. Retrieved from https://www.americanbar.org/groups/crsj/publications/human_rights_magazine_home/the-truth-about-science/politicization-of-science/
[19] Pan, I. W., Oeffinger, K. C., & Shih, Y. T. (2022). Cost-sharing and out-of-pocket cost for women who received MRI for breast cancer screening. Journal of the National Cancer Institute, 114(2), 254–262. https://doi.org/10.1093/jnci/djab150
[20] Yao, R., Zhang, W., Evans, R., Cao, G., Rui, T., & Shen, L. (2022). Inequities in health care services caused by the adoption of digital health technologies: Scoping review. Journal of Medical Internet Research, 24(3), e34144. https://doi.org/10.2196/34144
Comments